Data analytics and insights have become indispensable tools for businesses in today’s digital age. With the proliferation of data generated by various sources such as social media, online transactions, and customer interactions, organizations are realizing the immense potential locked within this data. By leveraging advanced analytics techniques, businesses can extract valuable insights that drive informed decision-making, optimize operations, and gain a competitive edge in the market. In this article, we will delve into the world of data analytics, exploring its concepts, methodologies, and the transformative impact it has on businesses across industries.
Understanding Data Analytics
Data analytics is the process of examining, cleansing, transforming, and modeling data to uncover meaningful patterns, draw conclusions, and drive business decisions. It involves a systematic approach that combines statistical analysis, predictive modeling, machine learning, and other techniques to extract insights from raw data. By applying data analytics, organizations can identify trends, discover correlations, and make data-driven predictions that inform strategic planning, resource allocation, and operational improvements.

What Is Data Analytics And Insights?
Data analytics is the process of examining large data sets to uncover hidden patterns and insights. The insights can be used to make better decisions, improve business performance, and understand customer behavior, many businesses now use data analytics to make informed decisions about where to allocate resources, how to price products and services, and what marketing campaigns to pursue.
Data analytics can also help you understand your customers better. By analyzing customer data, you can identify trends and preferences, and then use that information to create a more tailored customer experience. Data analytics is a powerful tool that can help businesses of all sizes make better decisions and improve their performance. If you’re not currently using data analytics, it’s worth considering.
What Is The Difference Between Data Analyst And Insights?

There is a big difference between data analysts and insights. Data analysts are responsible for taking data and turning it in to information. They clean and organize data, figure out what it means, and develop ways to use it to help a company make better decisions. Insights, on the other hand, are the end goal of data analysis. They are the important findings that data analysts uncover, and they can be used to help a company make better decisions, understand their customers, and compete more effectively.
Data analysts are essential to any company that wants to make the most of its data. By taking data and turning it in to information, they make it possible for companies to understand what is happening in their business and to make decisions based on that understanding. Insights, on the other hand, are the valuable findings that data analysts uncover. They can be used to help a company make better decisions, understand their customers, and compete more effectively.
What Is An Example Of Insight In Data Analytics?
Insight in data analytics is the ability to see patterns and trends in data that are not immediately obvious. This can help businesses to make better decisions by understanding what is happening in their data and how it affects their business.
For example, a business might use data analytics to understand how their website is being used. They might see that a particular page on their website is getting a lot of traffic, and they might decide to change that page to reflect what their customers are looking for.
Another example of insight in data analytics might be a business that sells products online. By understanding how customers are browsing their website and what products they are looking at, the business can make better decisions about what products to stock and how to market them.
How Do I Get Insights For Data Analytics?
The first step is to identify the business question you want to answer. The second step is to identify the data you need to answer that question. The third step is to find the right data analytics tool to help you analyze that data.
For example, suppose you want to know how many people visit your website each day. You would need to identify the business question you want to answer (in this case, how many people visit your website each day) and then identify the data you need to answer that question. In order to answer the question, “How many people visit your website each day?” you would need to collect data on the number of website visits each day.
There are a variety of data analytics tools available, and the right tool for you will depend on the type of data you are trying to analyze. The fourth step is to collect the data and analyze it. Once you have collected the data, you can use the data analytics tool to analyze it and answer your question. The fifth step is to take action based on the data. After you have analyzed the data, you should take action based on what you have learned. This could involve making changes to your website or marketing strategy.
The Data Analytics Process
The data analytics process typically involves several stages, including data collection, data preparation, analysis, and interpretation. Let’s explore each stage in detail:
- 1. Data Collection: This stage involves gathering data from various sources, both internal and external to the organization. Internal sources may include transactional databases, customer records, and operational systems, while external sources may include social media platforms, market research reports, and industry data. It is essential to ensure that the collected data is accurate, comprehensive, and relevant to the analysis objectives.
- 2. Data Preparation: Raw data often requires preprocessing to ensure its quality and compatibility for analysis. This stage involves cleaning the data to remove errors, inconsistencies, and duplicates. Additionally, data may need to be transformed, such as converting categorical variables into numerical formats or aggregating data into meaningful units. Data preparation also involves addressing missing values and outliers, which can significantly impact the accuracy of the analysis.
- 3. Analysis: Once the data is prepared, various statistical and analytical techniques are applied to extract insights. This stage may involve exploratory data analysis, which aims to understand the data’s characteristics, patterns, and distributions. It may also include hypothesis testing, regression analysis, clustering, classification, or predictive modeling, depending on the analysis goals.
- 4. Interpretation: The final stage of data analytics involves interpreting the results to derive actionable insights. Data visualization techniques, such as charts, graphs, and dashboards, are often employed to present the findings in a concise and comprehensible manner. Interpretation is a crucial step as it bridges the gap between raw data and actionable recommendations, enabling decision-makers to make informed choices based on the insights derived from the analysis.
Applications of Data Analytics

Data analytics finds applications across various industries and business functions. Some common use cases include:
1. Marketing and Customer Analytics: Data analytics helps businesses understand customer behavior, preferences, and purchasing patterns. By analyzing customer data, organizations can segment their target audience, personalize marketing campaigns, and optimize customer acquisition and retention strategies.
2. Operations and Supply Chain Management: Data analytics enables businesses to optimize their supply chain by analyzing data related to inventory levels, production schedules, transportation routes, and demand patterns. By identifying bottlenecks, inefficiencies, and areas for improvement, organizations can streamline their operations, reduce costs, and enhance overall productivity.
3. Risk Management and Fraud Detection: Data analytics plays a crucial role in identifying potential risks and detecting fraudulent activities. By analyzing historical data, organizations can build predictive models that identify suspicious patterns, enabling them to mitigate risks and prevent fraud.
4. Financial Analysis and Forecasting: Financial institutions leverage data analytics to gain insights into market trends, assess investment opportunities, and predict financial outcomes. By analyzing historical market data and economic indicators, organizations can make informed decisions regarding investments, portfolio management, and risk assessment.
Challenges and Opportunities
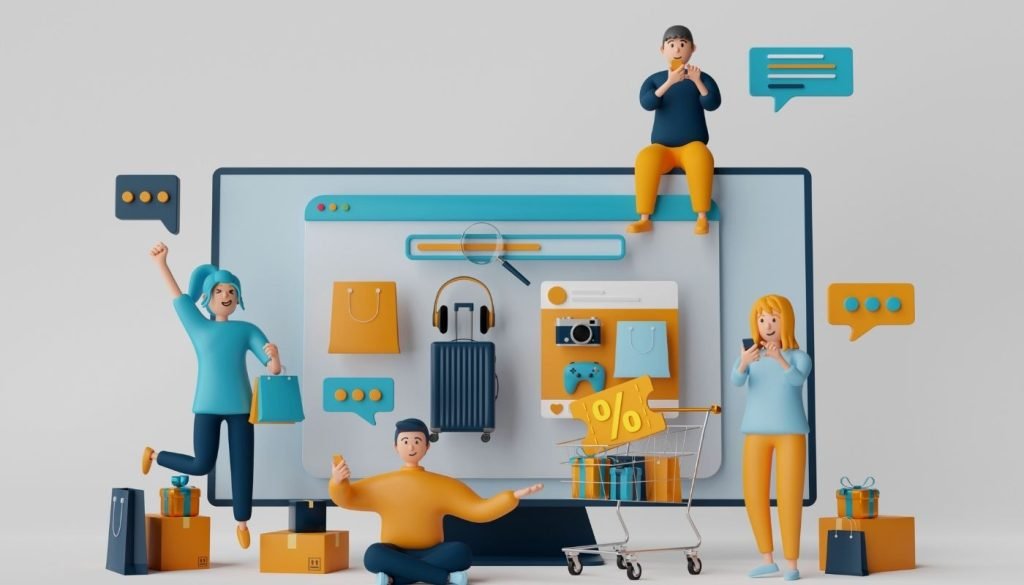
While data analytics offers immense opportunities, it also presents several challenges that organizations must overcome to harness its full potential. Some key challenges include:
1. Data Quality and Integration: Ensuring the quality and integrity of data is crucial for accurate analysis. Organizations often struggle with data silos, where data is dispersed across multiple systems and lacks integration. Data cleansing and integration processes can be time-consuming and resource-intensive.
2. Skill Gap: Data analytics requires a unique skill set encompassing statistics, programming, data visualization, and domain knowledge. Many organizations face challenges in finding and retaining skilled data analysts and data scientists who can effectively analyze and interpret data.
3. Data Privacy and security: As organizations collect and store vast amounts of sensitive data, ensuring data privacy and security becomes paramount. Compliance with data protection regulations and implementing robust security measures to safeguard data from unauthorized access or breaches pose significant challenges.
Data Analytics And Insights Opportunities

Despite these challenges, organizations that successfully embrace data analytics can unlock numerous opportunities, including:
1. Competitive Advantage: Data analytics enables organizations to gain a competitive edge by empowering them with data-driven insights for strategic decision-making. By understanding market trends, customer preferences, and competitor behavior, organizations can outperform their peers and adapt to changing market dynamics quickly.
2. Process Optimization: By analyzing operational data, organizations can identify process inefficiencies, bottlenecks, and areas for improvement. Data analytics helps streamline operations, reduce costs, and enhance overall efficiency.
3. Personalized Customer Experience: Data analytics enables organizations to deliver personalized experiences to customers. By analyzing customer data, businesses can understand individual preferences, anticipate their needs, and provide tailored recommendations and offerings, resulting in higher customer satisfaction and loyalty.
4. Innovation and Product Development: Data analytics can fuel innovation by providing insights into emerging trends and customer demands. Organizations can leverage data analytics to identify unmet needs, develop new products, and enhance existing offerings, driving innovation and market growth.
Conclusion
Data analytics and insights have emerged as powerful tools that drive informed decision-making, enhance operational efficiency, and unlock growth opportunities for businesses. By harnessing the vast amounts of data generated daily, organizations can gain a competitive advantage, optimize processes, and deliver personalized experiences to customers. However, realizing the full potential of data analytics requires organizations to overcome challenges related to data quality, skill gaps, and data privacy. With the right strategies, technologies, and talent, organizations can leverage data analytics to transform their operations, innovate, and thrive in the digital era.